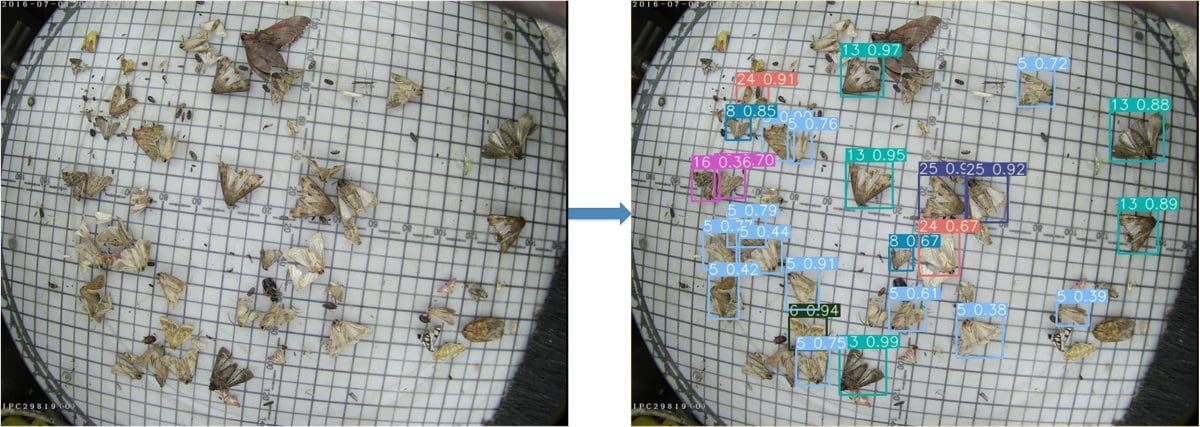
Researchers have added features to the YOLOv3 machine-learning platform to identify insects.
WESTERN Australian scientists have trained artificial intelligence to identify insect pests faster than anything else in the techno-sphere, creating a world-beating insect detector.
The researchers from Murdoch University and the WA Government achieved an ID accuracy of 72.1 percent, which beats existing techniques, while achieving a fast detection rate of 63.8 images per second.
They used a machine-learning platform called YOLOv3, a network that acts as an efficient and simple detector which learns to identify objects of different sizes.
They added a feature that allowed the detector to learn differing spatial elements.
“Traditionally, visual inspection and manual counting were done to acquire information on insect populations,” lead researcher and information technology expert Professor Ferdous Sohel said.
“However, these methods are labour intensive, time consuming and potentially inconsistent due to the human factor.
“With the rapid development of machine learning and deep-learning techniques, automatic detection of agricultural insects is now feasible.
“Developing multi-insect detection strategies has become an essential part of pest management.”
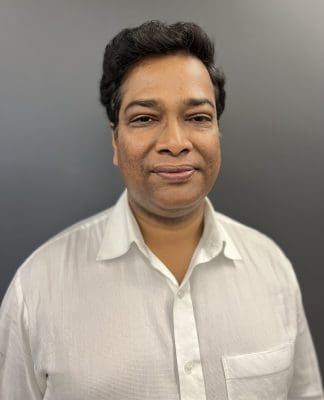
Professor Ferdous Sohel.
Professor Sohel said the team’s aim was to achieve a high-performance agricultural insect detector using an enhanced AI machine-learning technique.
They developed an algorithm using a dataset of 25,878 images of insects including beetles, weevils, and moths.
The researchers recorded a mean average precision rate of 71.6pc and then, by tackling insect images with complicated background, they lifted the detection accuracy by a further 3pc.
“From a computer algorithm point of view, insect detection from imagery presents a problem because they are so small.
“Detection of tiny objects in large datasets is challenging due to small resolution of the insects in an image, and other nuisances such as occlusion (when objects come too close or overlap others), noise and lack of features and texture.”
Experimental results show a significant improvement in insect detection accuracy compared to other state-of-the-art object detectors.
Additionally, their development had minimal impact on the processing speed.
“Also, it is applicable for real-time automatic insect detection from images on a large multi-scale agricultural pest dataset.”
There were some drawbacks.
The model’s performance was poorer for images containing overlapping small insects.
Also, the machine had trouble identifying insects of similar colour and shape, and differences in size and scale caused an imbalance in the dataset, which decreases accuracy.
“In future, we plan to address the detection of overlapped insects, insects with high similarity and scale variation.
“And more research is required to increase the accuracy of detection in challenging conditions.”
Grain Central: Get our free news straight to your inbox – Click here
HAVE YOUR SAY