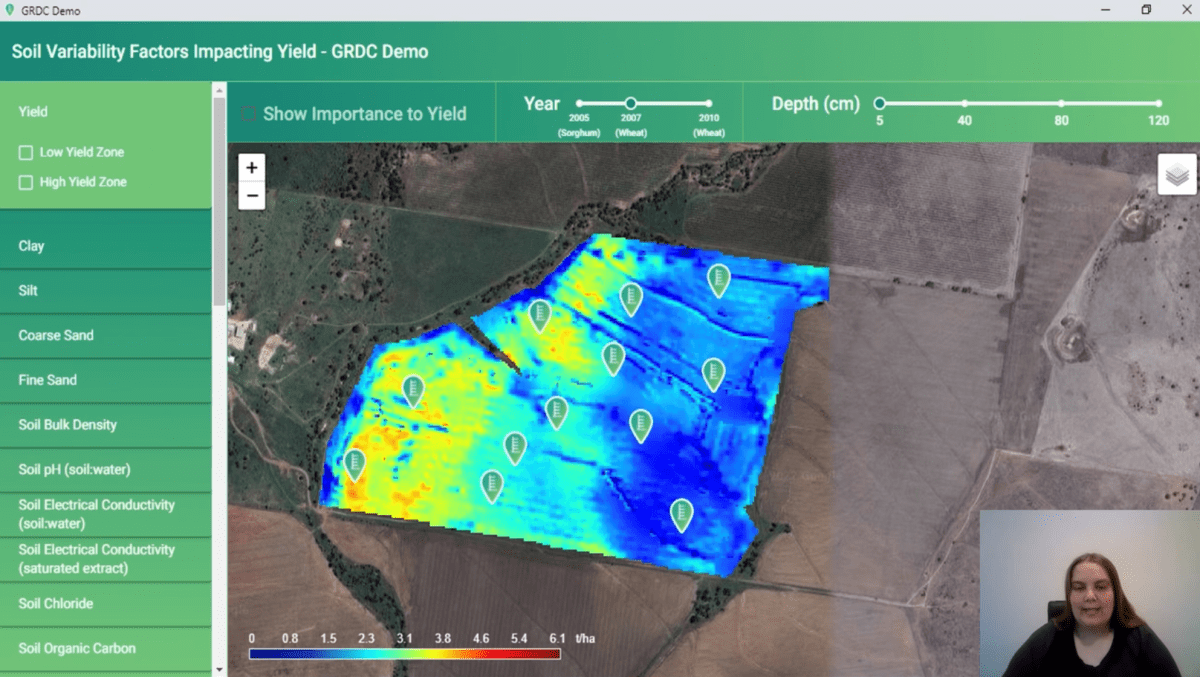
SARDI scientist Rhiannon Schilling showcases a demonstration application created by using paddock data and machine learning models. Photo: ABARES
PINPOINTING the cause of paddock-yield variability using large data sets and innovative machine-learning models is the focus of a project led by the University of Adelaide and funded by the Grains Research and Development Corporation (GRDC).
South Australian Research and Development Institute (SARDI) agriculture scientist Rhiannon Schilling gave an update of the project at ABARES Outlook online this week.
Ms Schilling said the research looked at the challenge of working out what is behind variability in crop growth and yield across paddocks.
“Often there has been a focus on improving grain yields of our varieties; but when we drive around and have a look at our paddocks, we can see that we are not always achieving uniform crop growth and yield across our paddocks,” Ms Schilling said.
“What we are wanting to do is use some of those historic mapping layers, such as, the soil, climate, topography and remote-sensing information to see if we can shift away from just zoning paddocks from low and high-yielding zones to really try to understand what was causing that variability in yield.”
Ms Schilling said the team collaborated with experts from the Australian Institute of Machine Learning to create a model which could interpret a large amount of data taken from a specific paddock and give the producer an insight on what is causing yield issues at a particular location.
“What we were able to do as part of the project was bring together our growers, agronomists and researchers to share data at that paddock scale and be able to use that to develop a relevant machine learning model,” she said.
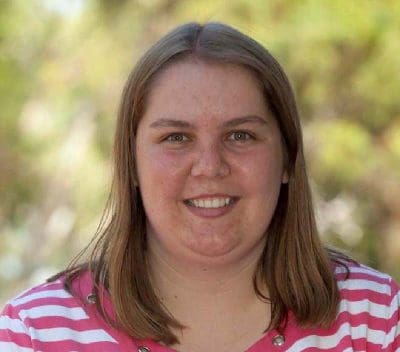
Rhiannon Schilling. Photo: University of Adelaide
“We have used those outputs from the machine learning to develop a demo app where we can now showcase what we have been able to observe.”
Ms Schilling said the app gives the user a visual depiction of multiple traits measured on the paddock and shows which ones have the most impact on yield.
“To take it one step further, what we can do with this information is to start to look through time at how important…traits were across growing seasons, how they vary, which ones were always important and which ones vary between seasons.”
She said the app can delve into a high level of detail from looking at specific values of a nutrient in the soil, at a particular soil depth and in a defined year.
Ms Schilling said the overall goal of the project is showcase how machine learning technology can be used to increase crop yields.
“If we can understand what is causing that variability in yield, we can then inform different management decisions and help to achieve a more uniform yields across those paddocks.
“We want to show what we can do with the machine-learning model and how it can be used by growers, agronomists and researchers going forward.”
Reduce data burden on farmers
Ms Schilling said researchers understood that growers were gathering large quantities of data, which takes up their time and resources.
As an example, she said the machine-learning model used 150 soil traits which highlighted the amount of information that can be captured from a paddock.
“With the model, we can identify the ones that are reoccurring as being important, so we can start focusing on them going forward and, with the others, not monitor them every year, but monitor them every now and then going forward.
“That will help reduce the time and investment and also that curation and storage of that data.”
Ms Schilling said deciding which trait or mapping layer was important varies paddock to paddock.
“Once you know what is driving that yield in those paddocks, then you can start to tailor which mapping layers you should be collecting more than others.”
She said it is still early days with the app and the model and researchers are now looking into how it can be better adapted to suit different soil types and regions.
Grain Central: Get our free news straight to your inbox – Click here
HAVE YOUR SAY